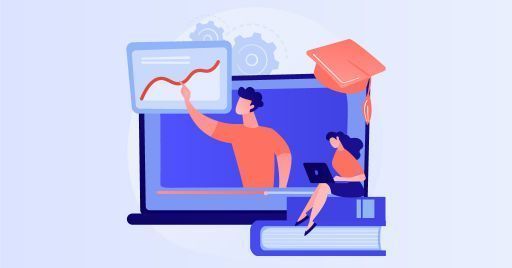
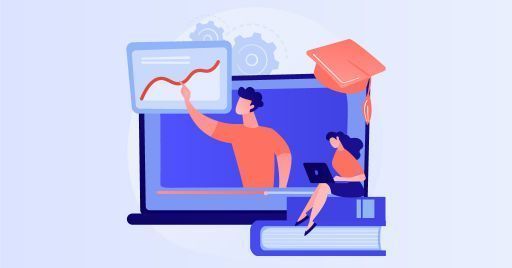
Predictive analytics to prevent dropout in online courses
Let’s discover how predictive analytics can help improve understanding of student behavior and address the risk of course dropouts.
Predictive analytics to prevent dropout in online courses
Let’s discover how predictive analytics can help improve understanding of student behavior and address the risk of course dropouts.
Predictive analytics offers advanced features to enhance the effectiveness of online education, utilizing data mining techniques, statistical modeling, and machine learning to analyze historical data and predict future behaviors and outcomes. In the context of online courses, it is essential for identifying students at risk of dropping out and intervening before it happens.
Implementing predictive analytics in online courses means improving the quality of education provided. Educators can use the insights obtained to adapt teaching materials and develop targeted teaching strategies. With this tool, eLearning becomes not only more reactive but also a deeply personalized and more effective learning environment.
Early identification of at-risk students
One of the most innovative aspects of predictive analytics in online courses is its ability to identify students at risk of dropping out early. This process relies on analyzing large datasets collected through Learning Management Systems (LMS), which continuously monitor various aspects of student behavior, such as access time to materials, interaction frequency with content, and assessment results.
Predictive analytics techniques detect patterns and trends in study approaches that may indicate impending difficulties. For example, a decrease in course interaction or a series of low scores on assessments could signal potential disengagement. With this data, interventions can be made before the student decides to drop out, offering personalized support and additional resources to reignite their engagement and interest. Educators can then adjust the pace and depth of the courses to meet individual needs, ensuring that each student receives the necessary attention and support to succeed.
Personalizing the learning path
Personalization of the learning path is another advantage of predictive analytics in online courses. Using detailed data on student behavior and performance, educators can create tailored learning experiences that adapt to each student’s individual needs and preferences.
Predictive analytics allows the detection of each student’s strengths and weaknesses through the analysis of their interactions with learning materials and exam results. This information is crucial for modulating content, adjusting the difficulty level of lessons, and providing additional resources. For example, if a student excels in a particular area, the system can automatically suggest more advanced content or supplementary challenges to maintain their interest.
Similarly, if the system identifies that a student is struggling with a certain topic, it can immediately offer support materials, review sessions, or modify the learning path to reduce frustration and prevent dropout. This form of adaptive learning ensures that each student progresses at their optimal pace, maximizing the effectiveness of the time spent on courses.
Feedback from predictive analytics and course modifications
An instructional designer can make several changes to an eLearning course to address a high dropout rate or low engagement rate. Here are some of the main changes:
Content personalization
Content personalization is based on the use of predictive analytics to tailor teaching materials to the individual needs and preferences of students. This strategy may involve adjusting the difficulty of assignments, modifying learning paths, and offering supplemental resources to students showing interest in specific areas. The benefits include increased engagement, greater student satisfaction, and ultimately a reduction in dropout rates, as the content becomes more relevant and stimulating for each student.
Introduction of interactive elements
Interactive elements, such as quizzes, polls, and simulations, can transform a passive learning experience into an active one, directly involving students in the educational process. Interactivity helps maintain students’ attention and continuously assess their learning, allowing timely corrections to the learning path.
Improving accessibility
Improving accessibility means making eLearning courses usable by students with different abilities and learning preferences. This can include offering content in multiple formats (text, audio, video), using subtitles, audio descriptions, and implementing web accessibility standards. Improving accessibility not only helps comply with legal regulations but ensures that all students have equal opportunities.
User interface optimization
A well-designed user interface significantly enhances the online learning experience. This includes intuitive navigation, clean layouts, and clear instructions that help students easily find what they need and focus on learning rather than figuring out how to use the platform.
Implementing immediate feedback
Providing immediate feedback helps students understand where they are going wrong and what they can do to improve, making them feel more supported and guided in their learning journey.
Increasing peer-to-peer support
Peer-to-peer support, such as discussion forums and study groups, can significantly increase student engagement. These spaces allow students to interact, discuss, and solve problems together, creating a learning community that fosters a sense of belonging and motivation. Additionally, peer learning can help clarify difficult concepts and offer different perspectives.
Optimizing resources and timely interventions
The effectiveness of predictive analytics extends to optimizing resources and timely interventions. This strategy allows for more effective resource allocation and prompt intervention when necessary, significantly improving operational efficiency and educational effectiveness.
With predictive analytics, large amounts of data generated by students can be analyzed to identify which teaching resources are most effective and which need improvement. This allows educators to focus time and investment on materials and activities that have been shown to increase engagement and performance, optimizing the use of available resources.
Timely, data-driven interventions can prevent the emergence of major issues, such as dropout. By early identification of signs of difficulty or disinterest, educators can implement personalized support strategies, transforming the educational environment into a more adaptive and responsive one.
Technologies and tools for predictive analytics
The effective implementation of predictive analytics in online courses heavily depends on the selection and use of appropriate technologies and tools. These advanced data science and machine learning tools are essential for processing and analyzing large volumes of data generated by students, allowing the prediction of future behaviors and outcomes.
Among the most commonly used technologies are Learning Management Systems (LMS), which integrate advanced analytics features to monitor student interactions with educational materials. These systems can track metrics such as time spent on content, access frequency, and assessment scores, providing a rich data foundation for predictive analysis.
Likewise, machine learning tools like Python and R are frequently used to develop predictive models that can identify patterns and trends in student data. Additionally, platforms like Tableau or Power BI are used to visualize analytical data, making the results easily interpretable for decision-makers. These visualizations can help communicate complex findings intuitively, supporting quick and informed decisions.
Ethical issues and data privacy
While educators and institutions use student data to improve the effectiveness of educational interventions, it is crucial to ensure that this information is handled with the utmost confidentiality and security.
The collection and use of data must be regulated by strict privacy policies in compliance with current regulations, such as the GDPR in the European Union, which imposes strict standards for the protection of personal data. Students must be informed about which data is collected and used, as well as the security measures adopted to protect their personal information.
Furthermore, predictive analytics must be used fairly and equitably, avoiding discrimination or biases that could arise from the misinterpretation of data or the use of inadequately calibrated algorithms. It is essential that predictive models be transparent and regularly reviewed to ensure they do not perpetuate pre-existing biases.
Only with transparent communication and respect for privacy will students feel secure and engaged in their online learning journey, confident that their data is used to support, not penalize, their educational progress.
Future prospects
As technology continues to evolve, the future prospects of predictive analytics appear even more promising, with new developments aimed at further refining personalized learning and educational intervention.
In the future, we can expect it to become even more integrated with artificial intelligence and machine learning technologies, enhancing the ability to predict student needs in real-time with greater precision. At the same time, increasing awareness of privacy and ethical issues will push for stricter regulatory developments and better practices in data handling. Transparency in algorithm use and personal data protection will become increasingly critical standards to safeguard student trust and well-being.